Bluetooth Low Energy
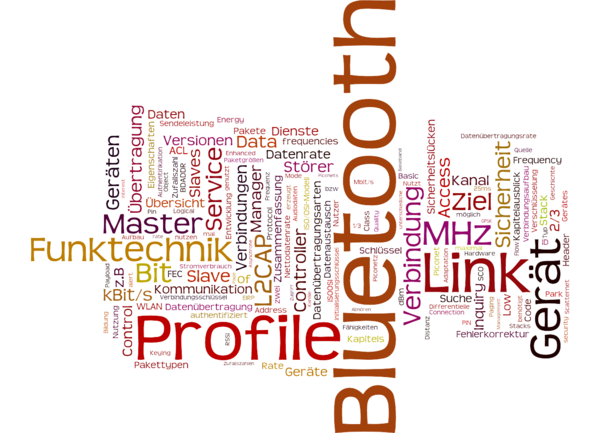
Smartphones have become for many users a device which accompanies them during the whole day. A consequence of this trend is the ubiquitous availability of Bluetooth and (with Standard 4.1) Bluetooth low energy. Bluetooth low energy devices are known for their inexpensive and energy preserving design (battery powered). Thus they are well-prepared for localization purposes in beacon scenarios.
However, Bluetooth Low Energy also offers plenty of opportunities for the Internet of Things (IoT) and Industry 4.0. Parallel to our Bluetooth localization efforts we also take a look at communication and interaction aspects of Bluetooth, e.g. in Smart Home Environments.
Received Signal Strength Indicator
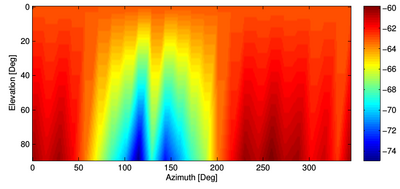
We measured the average emitted power of a beacon as a function of the azimuth and the elevation angle in our antenna laboratory using an Android smartphone. The results are depicted in the left figure. While these particular results certainly depend on the beacon and smartphone used, and will be different if other devices are employed, it can be observed in general that the radiation pattern of BLE beacons is not omnidirecional. This fact will have a significant impact on the localization accuracy.
Position accuracy
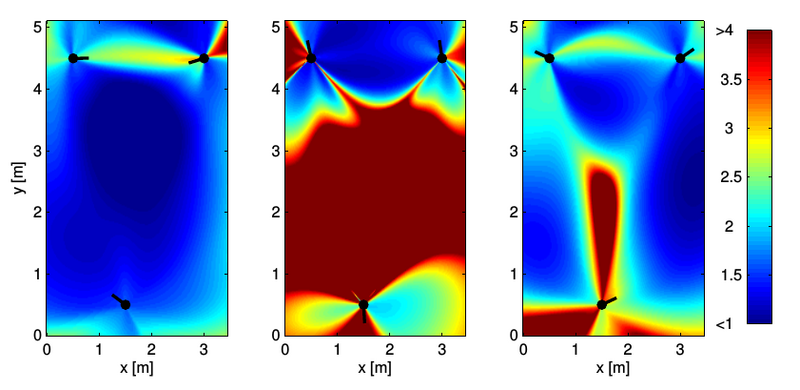
For the results in the right figure we kept the positions of the three beacons fixed and drew the orientation of each beacon uniformly at random. For each configuration we calculated the root CRLB averaged over 150 positions placed on a regular grid in the room of size given above. The best setup achieves an RMSE of less than 1.5 m while the worst ones exceed an RMSE of 4 m. These results clearly show the potential benefits of integrating direction information into the beacon network planning process.
Triangulation vs. Grid-based Localization
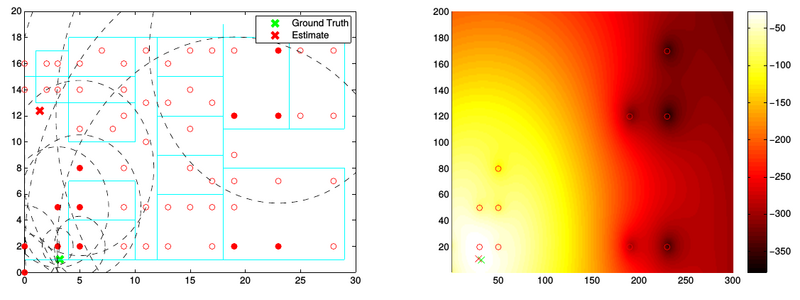
The task of localizing a smartphone in a building can be solved in various ways. The most simplest one may be triangulation, where the signal strength information is first transformed into distance estimates between smartphone and beacons and, afterwards, a least-squares approach delivers the position estimate. In ideal environments with only slightly erronous measurements the approach works well.
However, in real environments BLE RSSI signal measurements tend to have large errors and thus triangulation will fail. In the upper example the system tries to combine the observations from different beacons, where a subset of beacons have very erronous oberservations. The result is large localization error. In comparison the result of a grid-based approach is depicted. Without the need of identifying the outliers the grid-based approach remains a robust estimator. Its core implementation models all signals as random variables and tries to estimate the most likely position given all observations.